Visualizing and Comparing Machine Learning Predictions to Improve Human-AI Teaming on the Example of Cell Lineage
Jiayi Hong -
Ross Maciejewski -
Alain Trubuil -
Tobias Isenberg -
Screen-reader Accessible PDF
Download preprint PDF
DOI: 10.1109/TVCG.2023.3302308
Room: Bayshore V
2024-10-17T13:06:00ZGMT-0600Change your timezone on the schedule page
2024-10-17T13:06:00Z
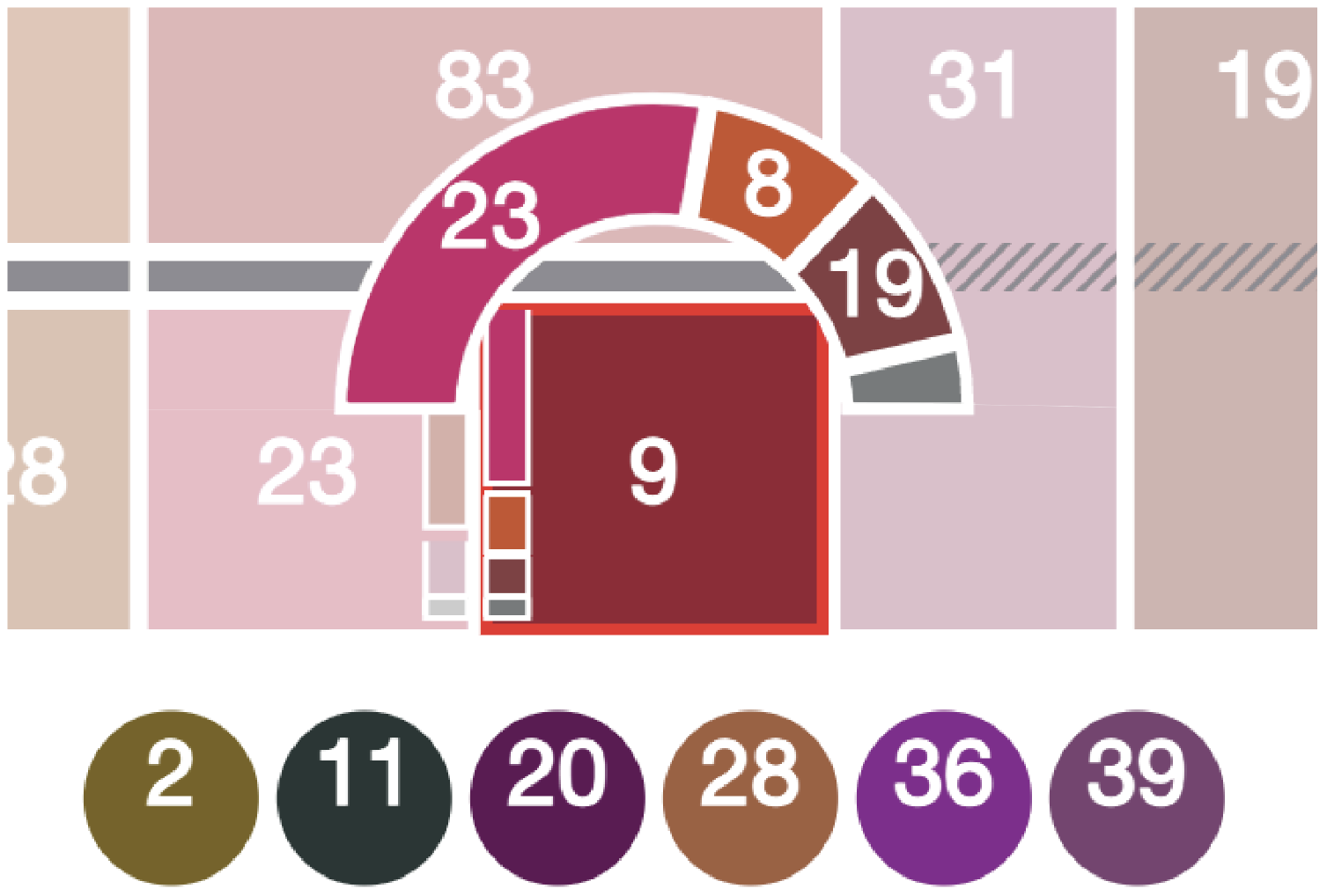
Fast forward
Full Video
Keywords
Visualization, visual analytics, machine learning, comparing ML predictions, human-AI teaming, plant biology, cell lineage
Abstract
We visualize the predictions of multiple machine learning models to help biologists as they interactively make decisions about cell lineage---the development of a (plant) embryo from a single ovum cell. Based on a confocal microscopy dataset, traditionally biologists manually constructed the cell lineage, starting from this observation and reasoning backward in time to establish their inheritance. To speed up this tedious process, we make use of machine learning (ML) models trained on a database of manually established cell lineages to assist the biologist in cell assignment. Most biologists, however, are not familiar with ML, nor is it clear to them which model best predicts the embryo's development. We thus have developed a visualization system that is designed to support biologists in exploring and comparing ML models, checking the model predictions, detecting possible ML model mistakes, and deciding on the most likely embryo development. To evaluate our proposed system, we deployed our interface with six biologists in an observational study. Our results show that the visual representations of machine learning are easily understandable, and our tool, LineageD+, could potentially increase biologists' working efficiency and enhance the understanding of embryos.