Confides: A Visual Analytics Solution for Automated Speech Recognition Analysis and Exploration
Sunwoo Ha - Washington University in St. Louis, St. Louis, United States
Chaehun Lim - Washington University in St. Louis, St. Louis, United States
R. Jordan Crouser - Smith College, Northampton, United States
Alvitta Ottley - Washington University in St. Louis, St. Louis, United States
Screen-reader Accessible PDF
Download preprint PDF
Download Supplemental Material
Room: Bayshore VI
2024-10-17T14:51:00ZGMT-0600Change your timezone on the schedule page
2024-10-17T14:51:00Z
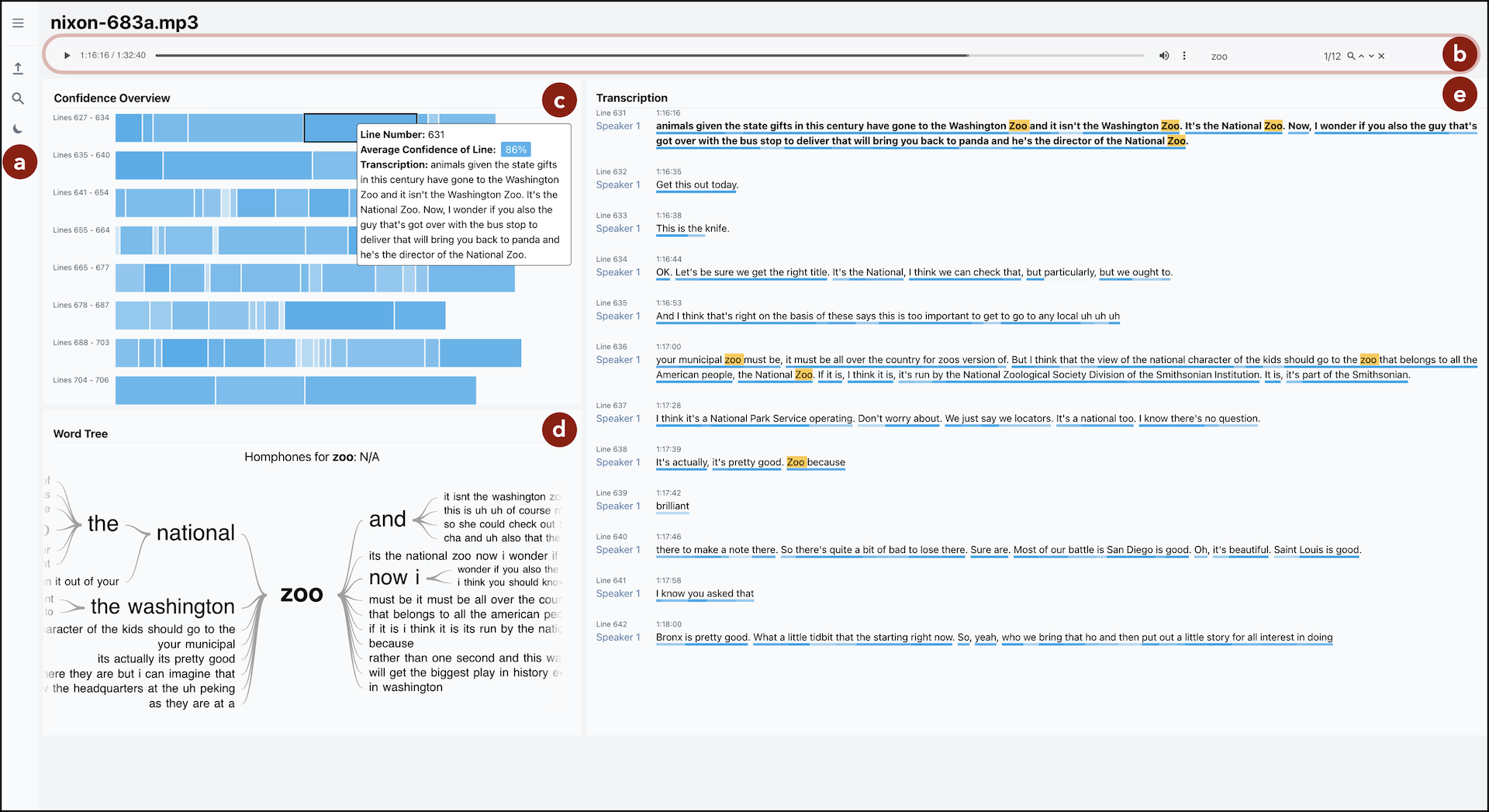
Fast forward
Full Video
Keywords
Visual analytics, confidence visualization, automatic speech recognition
Abstract
Confidence scores of automatic speech recognition (ASR) outputs are often inadequately communicated, preventing its seamless integration into analytical workflows. In this paper, we introduce Confides, a visual analytic system developed in collaboration with intelligence analysts to address this issue. Confides aims to aid exploration and post-AI-transcription editing by visually representing the confidence associated with the transcription. We demonstrate how our tool can assist intelligence analysts who use ASR outputs in their analytical and exploratory tasks and how it can help mitigate misinterpretation of crucial information. We also discuss opportunities for improving textual data cleaning and model transparency for human-machine collaboration.