FCNR: Fast Compressive Neural Representation of Visualization Images
Yunfei Lu - University of Notre Dame, Notre Dame, United States
Pengfei Gu - University of Notre Dame, Notre Dame, United States
Chaoli Wang - University of Notre Dame, Notre Dame, United States
Screen-reader Accessible PDF
Download preprint PDF
Download Supplemental Material
Room: Bayshore VI
2024-10-16T18:21:00ZGMT-0600Change your timezone on the schedule page
2024-10-16T18:21:00Z
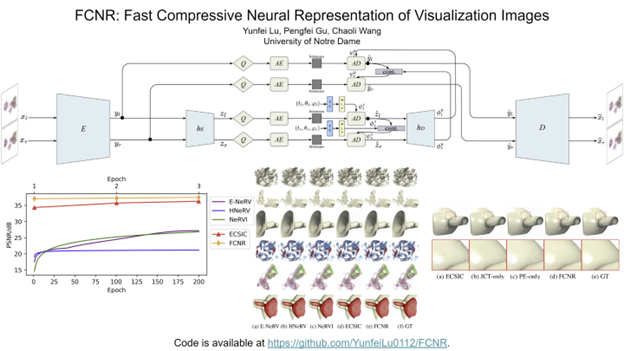
Fast forward
Full Video
Keywords
Machine Learning Techniques, Image and Video Data
Abstract
We present FCNR, a fast compressive neural representation for tens of thousands of visualization images under varying viewpoints and timesteps. The existing NeRVI solution, albeit enjoying a high compression ratio, incurs slow speeds in encoding and decoding. Built on the recent advances in stereo image compression, FCNR assimilates stereo context modules and joint context transfer modules to compress image pairs. Our solution significantly improves encoding and decoding speed while maintaining high reconstruction quality and satisfying compression ratio. To demonstrate its effectiveness, we compare FCNR with state-of-the-art neural compression methods, including E-NeRV, HNeRV, NeRVI, and ECSIC. The source code can be found at https://github.com/YunfeiLu0112/FCNR.