Regularized Multi-Decoder Ensemble for an Error-Aware Scene Representation Network
Tianyu Xiong - The Ohio State University, Columbus, United States
Skylar Wolfgang Wurster - The Ohio State University, Columbus, United States
Hanqi Guo - The Ohio State University, Columbus, United States. Argonne National Laboratory, Lemont, United States
Tom Peterka - Argonne National Laboratory, Lemont, United States
Han-Wei Shen - The Ohio State University , Columbus , United States
Room: Bayshore I
2024-10-16T13:30:00ZGMT-0600Change your timezone on the schedule page
2024-10-16T13:30:00Z
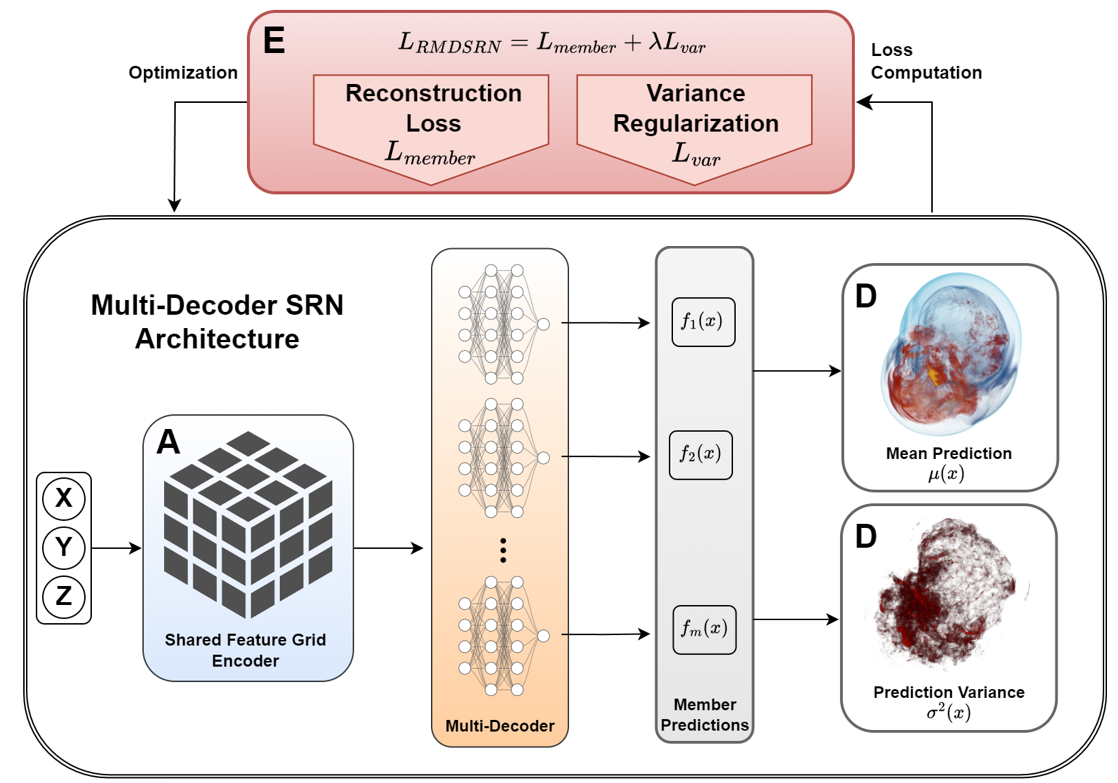
Fast forward
Full Video
Keywords
Scene representation network, deep learning, scientific visualization, ensemble learning
Abstract
Feature grid Scene Representation Networks (SRNs) have been applied to scientific data as compact functional surrogates for analysis and visualization. As SRNs are black-box lossy data representations, assessing the prediction quality is critical for scientific visualization applications to ensure that scientists can trust the information being visualized. Currently, existing architectures do not support inference time reconstruction quality assessment, as coordinate-level errors cannot be evaluated in the absence of ground truth data. By employing the uncertain neural network architecture in feature grid SRNs, we obtain prediction variances during inference time to facilitate confidence-aware data reconstruction. Specifically, we propose a parameter-efficient multi-decoder SRN (MDSRN) architecture consisting of a shared feature grid with multiple lightweight multi-layer perceptron decoders. MDSRN can generate a set of plausible predictions for a given input coordinate to compute the mean as the prediction of the multi-decoder ensemble and the variance as a confidence score. The coordinate-level variance can be rendered along with the data to inform the reconstruction quality, or be integrated into uncertainty-aware volume visualization algorithms. To prevent the misalignment between the quantified variance and the prediction quality, we propose a novel variance regularization loss for ensemble learning that promotes the Regularized multi-decoder SRN (RMDSRN) to obtain a more reliable variance that correlates closely to the true model error. We comprehensively evaluate the quality of variance quantification and data reconstruction of Monte Carlo Dropout (MCD), Mean Field Variational Inference (MFVI), Deep Ensemble (DE), and Predicting Variance (PV) in comparison with our proposed MDSRN and RMDSRN applied to state-of-the-art feature grid SRNs across diverse scalar field datasets. We demonstrate that RMDSRN attains the most accurate data reconstruction and competitive variance-error correlation among uncertain SRNs under the same neural network parameter budgets. Furthermore, we present an adaptation of uncertainty-aware volume rendering and shed light on the potential of incorporating uncertain predictions in improving the quality of volume rendering for uncertain SRNs. Through ablation studies on the regularization strength and decoder count, we show that MDSRN and RMDSRN are expected to perform sufficiently well with a default configuration without requiring customized hyperparameter settings for different datasets.