How Aligned are Human Chart Takeaways and LLM Predictions? A Case Study on Bar Charts with Varying Layouts
Huichen Will Wang - University of Washington, Seattle, United States
Jane Hoffswell - Adobe Research, Seattle, United States
Sao Myat Thazin Thane - University of Massachusetts Amherst, Amherst, United States
Victor S. Bursztyn - Adobe Research, San Jose, United States
Cindy Xiong Bearfield - Georgia Tech, Atlanta, United States
Room: Bayshore V
2024-10-18T13:18:00ZGMT-0600Change your timezone on the schedule page
2024-10-18T13:18:00Z
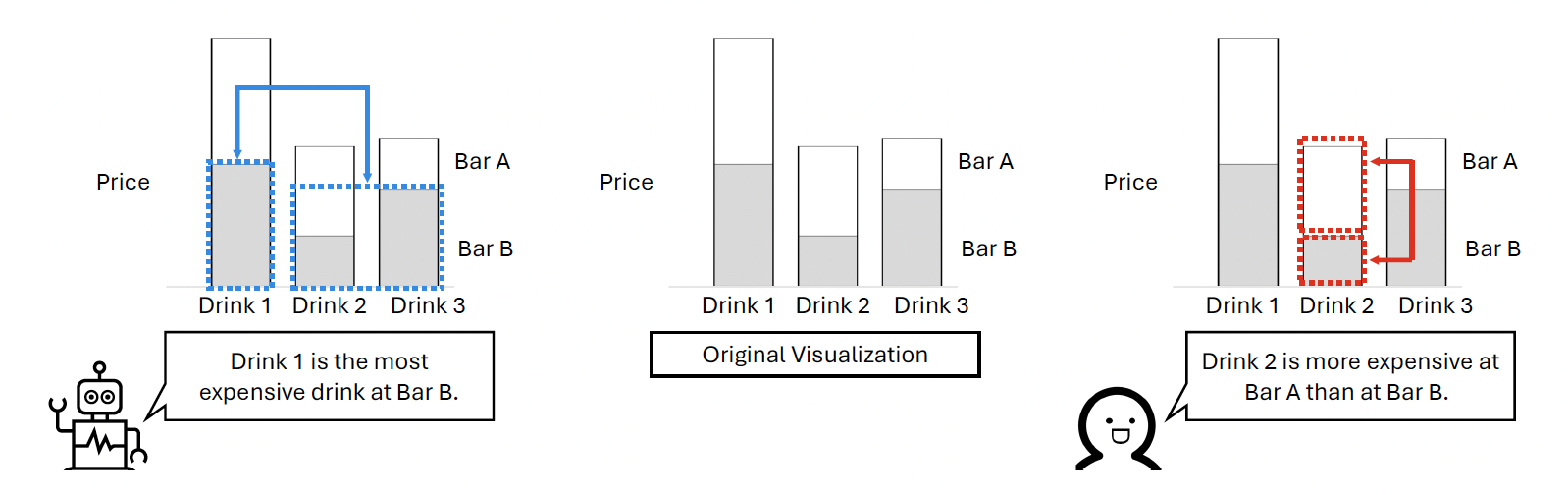
Fast forward
Full Video
Keywords
Visualization, Graphical Perception, Large Language Models
Abstract
Large Language Models (LLMs) have been adopted for a variety of visualizations tasks, but how far are we from perceptually aware LLMs that can predict human takeaways? Graphical perception literature has shown that human chart takeaways are sensitive to visualization design choices, such as spatial layouts. In this work, we examine the extent to which LLMs exhibit such sensitivity when generating takeaways, using bar charts with varying spatial layouts as a case study. We conducted three experiments and tested four common bar chart layouts: vertically juxtaposed, horizontally juxtaposed, overlaid, and stacked. In Experiment 1, we identified the optimal configurations to generate meaningful chart takeaways by testing four LLMs, two temperature settings, nine chart specifications, and two prompting strategies. We found that even state-of-the-art LLMs struggled to generate semantically diverse and factually accurate takeaways. In Experiment 2, we used the optimal configurations to generate 30 chart takeaways each for eight visualizations across four layouts and two datasets in both zero-shot and one-shot settings. Compared to human takeaways, we found that the takeaways LLMs generated often did not match the types of comparisons made by humans. In Experiment 3, we examined the effect of chart context and data on LLM takeaways. We found that LLMs, unlike humans, exhibited variation in takeaway comparison types for different bar charts using the same bar layout. Overall, our case study evaluates the ability of LLMs to emulate human interpretations of data and points to challenges and opportunities in using LLMs to predict human chart takeaways.