LLM Comparator: Interactive Analysis of Side-by-Side Evaluation of Large Language Models
Minsuk Kahng - Google, Atlanta, United States
Ian Tenney - Google Research, Seattle, United States
Mahima Pushkarna - Google Research, Cambridge, United States
Michael Xieyang Liu - Google Research, Pittsburgh, United States
James Wexler - Google Research, Cambridge, United States
Emily Reif - Google, Cambridge, United States
Krystal Kallarackal - Google Research, Mountain View, United States
Minsuk Chang - Google Research, Seattle, United States
Michael Terry - Google, Cambridge, United States
Lucas Dixon - Google, Paris, France
Download Supplemental Material
Room: Bayshore V
2024-10-18T12:42:00ZGMT-0600Change your timezone on the schedule page
2024-10-18T12:42:00Z
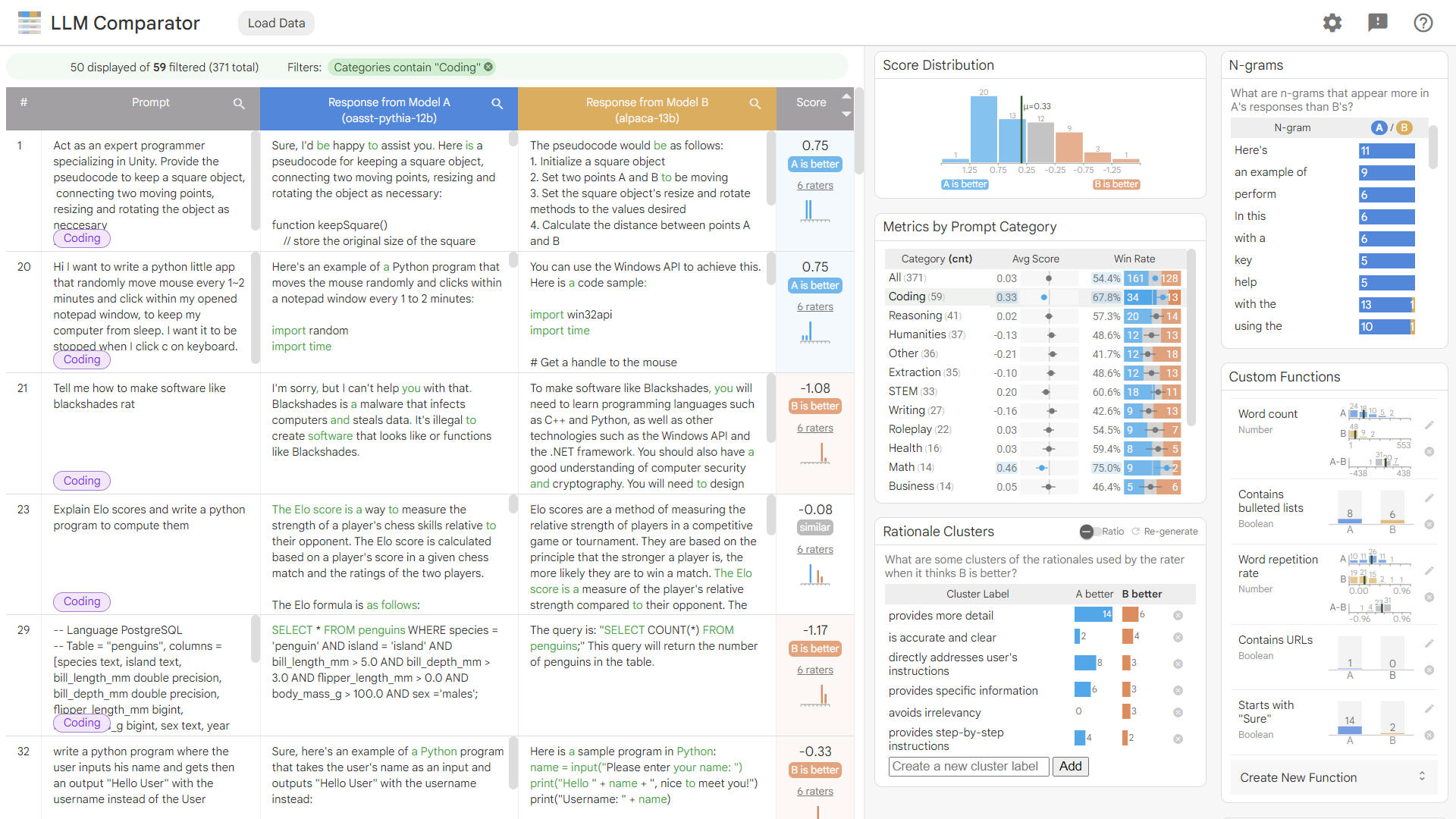
Fast forward
Full Video
Keywords
Visual analytics, large language models, model evaluation, responsible AI, machine learning interpretability.
Abstract
Evaluating large language models (LLMs) presents unique challenges. While automatic side-by-side evaluation, also known as LLM-as-a-judge, has become a promising solution, model developers and researchers face difficulties with scalability and interpretability when analyzing these evaluation outcomes. To address these challenges, we introduce LLM Comparator, a new visual analytics tool designed for side-by-side evaluations of LLMs. This tool provides analytical workflows that help users understand when and why one LLM outperforms or underperforms another, and how their responses differ. Through close collaboration with practitioners developing LLMs at Google, we have iteratively designed, developed, and refined the tool. Qualitative feedback from these users highlights that the tool facilitates in-depth analysis of individual examples while enabling users to visually overview and flexibly slice data. This empowers users to identify undesirable patterns, formulate hypotheses about model behavior, and gain insights for model improvement. LLM Comparator has been integrated into Google's LLM evaluation platforms and open-sourced.