DITTO: A Visual Digital Twin for Interventions and Temporal Treatment Outcomes in Head and Neck Cancer
Andrew Wentzel - University of Illinois at Chicago, Chicago, United States
Serageldin Attia - University of Houston, Houston, United States
Xinhua Zhang - University of Illinois Chicago, Chicago, United States
Guadalupe Canahuate - University of Iowa, Iowa City, United States
Clifton David Fuller - University of Texas, Houston, United States
G. Elisabeta Marai - University of Illinois at Chicago, Chicago, United States
Download preprint PDF
Download Supplemental Material
Room: Bayshore V
2024-10-17T18:45:00ZGMT-0600Change your timezone on the schedule page
2024-10-17T18:45:00Z
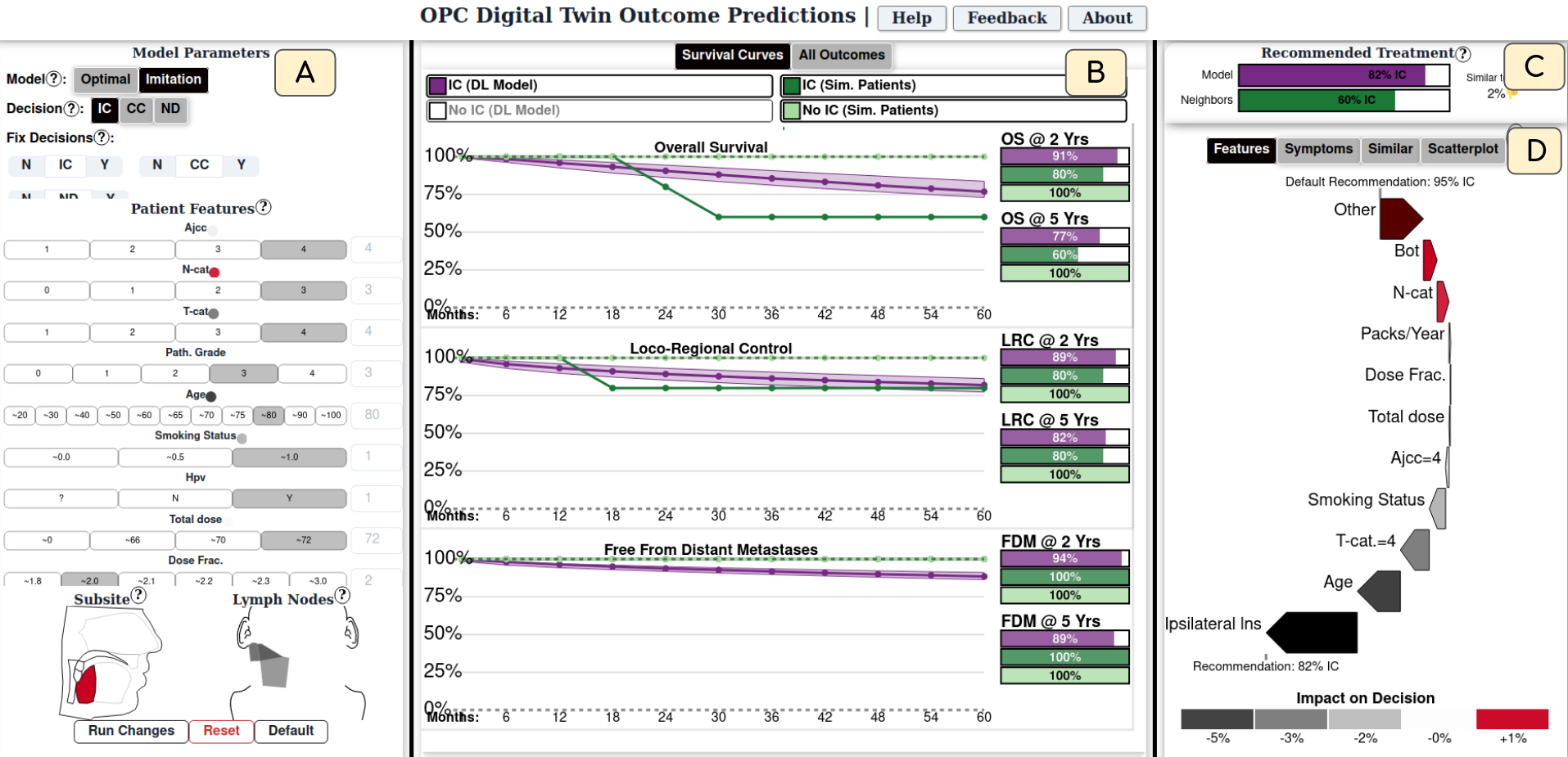
Fast forward
Full Video
Keywords
Medicine; Machine Learning; Application Domains; High Dimensional data; Spatial Data; Activity Centered Design
Abstract
Digital twin models are of high interest to Head and Neck Cancer (HNC) oncologists, who have to navigate a series of complex treatment decisions that weigh the efficacy of tumor control against toxicity and mortality risks. Evaluating individual risk profiles necessitates a deeper understanding of the interplay between different factors such as patient health, spatial tumor location and spread, and risk of subsequent toxicities that can not be adequately captured through simple heuristics. To support clinicians in better understanding tradeoffs when deciding on treatment courses, we developed DITTO, a digital-twin and visual computing system that allows clinicians to analyze detailed risk profiles for each patient, and decide on a treatment plan. DITTO relies on a sequential Deep Reinforcement Learning digital twin (DT) to deliver personalized risk of both long-term and short-term disease outcome and toxicity risk for HNC patients. Based on a participatory collaborative design alongside oncologists, we also implement several visual explainability methods to promote clinical trust and encourage healthy skepticism when using our system. We evaluate the efficacy of DITTO through quantitative evaluation of performance and case studies with qualitative feedback. Finally, we discuss design lessons for developing clinical visual XAI applications for clinical end users.